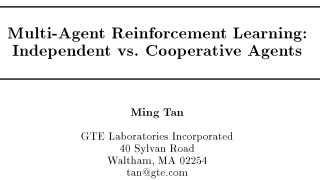
From the Abstract:
Intelligent human agents exist in a cooperative social environment that facilitates learning. They learn not only by trialand -error, but also through cooperation by sharing instantaneous information, episodic experience, and learned knowledge. The key investigations of this paper are, "Given the same number of reinforcement learning agents, will cooperative agents outperform independent agents who do not communicate during learning?" and "What is the price for such cooperation?" Using independent agents as a benchmark, cooperative agents are studied in following ways: (1) sharing sensation, (2) sharing episodes, and (3) sharing learned policies. This paper shows that (a) additional sensation from another agent is beneficial if it can be used efficiently, (b) sharing learned policies or episodes among agents speeds up learning at the cost of communication, and (c) for joint tasks, agents engaging in partnership can significantly outperform independent agents although they may learn slowly in the beginning. These tradeoffs are not just limited to multi-agent reinforcement learning.