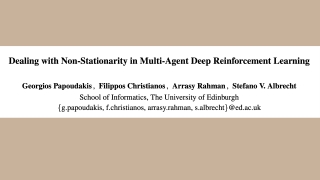
From the abstract
Recent developments in deep reinforcement learning are concerned with creating decision-making agents which can perform well in various complex domains. A particular approach which has received increasing attention is multi-agent reinforcement learning, in which multiple agents learn concurrently to coordi- nate their actions. In such multi-agent environments, additional learning problems arise due to the contin- ually changing decision-making policies of agents. This paper surveys recent works that address the non-stationarity problem in multi-agent deep rein- forcement learning. The surveyed methods range from modifications in the training procedure, such as centralized training, to learning representations of the opponent’s policy, meta-learning, commu- nication, and decentralized learning. The survey concludes with a list of open problems and possible lines of future research.